Linear Interpolation#
The simplest interpolation we can imagine is doing connect-the-dots, and simply connecting our data samples with straight lines.
As we see, this only gets the correct values at the end points (for a general function), but it has the nice property that it does not introduce any new minima or maxima.
Given some N points sampling a function, \((x_i, f_i)\), the interpolant connecting the points \(i\) and \(i+1\) is:
for \(x_i \le x \le x_{i+1}\)
For N points, we have \(N-1\) interpolants.
Piecewise interpolation#
This is an example of piecewise interpolation. We don’t try to fit all of the points with a single polynomial, but instead fit a subset of points with a low-order polynomial (in this case, a line).
Example
Let’s write a function to tabulate \(f(x) = x \sin(x)\) on \([0, 5]\) and then use linear interpolation to get the function values.
How many function samples do we need to get a 1% error?
import numpy as np
import matplotlib.pyplot as plt
def f(x):
return x * np.sin(x)
def sample_function(func, npts, *, xmin=0.0, xmax=10.0):
xx = np.linspace(xmin, xmax, npts)
return xx, func(xx)
Let’s look at this function and 10 samples
fig, ax = plt.subplots()
xmin = 0.0
xmax = 10.0
N = 10
x_fine = np.linspace(xmin, xmax, 1000)
x_sample, f_sample = sample_function(f, N, xmin=xmin, xmax=xmax)
ax.plot(x_fine, f(x_fine), label="true function")
ax.plot(x_sample, f_sample, marker="o", ls=":", label="linear interpolation")
ax.legend()
<matplotlib.legend.Legend at 0x7f3ec23b5610>
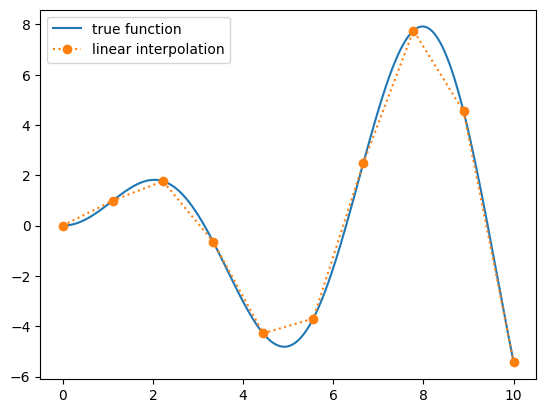
Now let’s define our interpolate function. This will take the location and values where the function is sampled, (xv
, fv
), and the location where we want to approximate the value of the underlying function, x0
.
def interpolate(x0, xv, fv):
# find first x[i] > x0
idx = np.argwhere(xv > x0)[0][0]
# we want to use this point and the one to the left
# we'll shift idx to point to the left point
idx = max(0, idx-1)
slope = (fv[idx+1] - fv[idx]) / (xv[idx+1] - xv[idx])
return slope * (x0 - xv[idx]) + fv[idx]
Let’s just look at \(x_0 = 4.5\) (this is near a minimum) and try different number of points and measure the error
x0 = 4.5
for n in [5, 10, 20, 40, 80, 160]:
xv, fv = sample_function(f, n)
finterp = interpolate(x0, xv, fv)
fexact = f(x0)
err = np.abs(finterp - fexact)
print(f"{n:4} points, error = {err}")
5 points, error = 0.8624245028923605
10 points, error = 0.14257394829953363
20 points, error = 0.1333081368280027
40 points, error = 0.03204962648664189
80 points, error = 0.007851522573614211
160 points, error = 0.0019427475977087383
In this case, after 80 points, our error is < 1%.
We also see that we appear to converge slightly better than 2nd order
C++ implementation#
Here’s a C++ version that follows the same ideas:
#include <iostream>
#include <cmath>
#include <vector>
#include <functional>
#include <print>
// our test function
double f(double x) {
return x * std::sin(x);
}
// a function that will produce N samples of the input func(x)
std::pair<std::vector<double>, std::vector<double>>
sample_function(std::function<double(double)> func,
int npts, double xmin, double xmax) {
std::vector<double> xv(npts, 0.0);
std::vector<double> fv(npts, 0.0);
double dx = (xmax - xmin) / static_cast<double>(npts - 1);
for (int i = 0; i < npts; ++i) {
xv[i] = xmin + static_cast<double>(i) * dx;
fv[i] = func(xv[i]);
}
return {xv, fv};
}
// the main linear intepolation function
double interpolate(double x0,
const std::vector<double>& xv,
const std::vector<double>& fv) {
// find the index i where xv[i] > x0
int idx = -1;
for (std::size_t i = 0; i < xv.size()-1; ++i) {
if (x0 > xv[i] && x0 < xv[i+1]) {
idx = i;
break;
}
}
if (idx == -1) {
std::cout << "x0 is not in range of xv" << std::endl;
}
double slope = (fv[idx+1] - fv[idx]) / (xv[idx+1] - xv[idx]);
return slope * (x0 - xv[idx]) + fv[idx];
}
// test driver
int main() {
// we want to find the N such that the error in our interpolation
// is < 1%. We'll just consider a bunch of different sizes for N
double xmin{0.0};
double xmax{10.0};
double x0{4.5};
for (auto N : {5, 10, 20, 40, 80, 160}) {
const auto [xv, fv] = sample_function(f, N, xmin, xmax);
auto finterp = interpolate(x0, xv, fv);
auto fexact = f(x0);
double err = std::abs(finterp - fexact);
std::println("{:4} points, error = {:7.5f}", N, err);
}
}