Adaptive RK4 Orbits#
import numpy as np
import matplotlib.pyplot as plt
We’ll use an implementation of the orbit problem with RK4 written as a module. The source (in both C++ and python) is here:
zingale/computational_astrophysics
import orbit_adaptive as orbit
Let’s first look at the source
%cat orbit_adaptive.py
Show code cell output
"""
orbital motion. We consider low mass objects orbiting the Sun. We
work in units of AU, yr, and solar masses. From Kepler's third law:
4 pi**2 a**3 = G M P**2
if a is in AU, P is in yr, and M is in solar masses, then
a**3 = P**2
and therefore
4 pi**2 = G
we work in coordinates with the Sun at the origin
This version implements adaptive timestepping
"""
import numpy as np
import matplotlib.pyplot as plt
# global parameters
GM = 4.0 * np.pi**2 # assuming M = 1 solar mass
class OrbitState:
# a container to hold the star positions
def __init__(self, x, y, u, v):
self.x = x
self.y = y
self.u = u
self.v = v
def __add__(self, other):
return OrbitState(self.x + other.x, self.y + other.y,
self.u + other.u, self.v + other.v)
def __sub__(self, other):
return OrbitState(self.x - other.x, self.y - other.y,
self.u - other.u, self.v - other.v)
def __mul__(self, other):
return OrbitState(other * self.x, other * self.y,
other * self.u, other * self.v)
def __rmul__(self, other):
return self.__mul__(other)
def __str__(self):
return f"{self.x:10.6f} {self.y:10.6f} {self.u:10.6f} {self.v:10.6f}"
class OrbitsRK4:
""" model the evolution of a single planet around the Sun"""
def __init__(self, a, e):
""" a = semi-major axis (in AU),
e = eccentricity """
self.a = a
self.e = e
x0 = 0.0 # start at x = 0 by definition
y0 = a*(1.0 - e) # start at perihelion
# perihelion velocity (see C&O Eq. 2.33 for ex)
u0 = -np.sqrt( (GM/a)* (1.0 + e) / (1.0 - e) )
v0 = 0.0
self.history = [OrbitState(x0, y0, u0, v0)]
self.time = [0.0]
self.n_reset = None
def npts(self):
""" return the number of integration points"""
return len(self.time)
def get_time(self, n):
""" return the time corresponding to step n"""
return self.time[n]
def kepler_period(self):
""" return the period of the orbit in yr """
return np.sqrt(self.a**3)
def final_R(self):
""" the radius at the final integration time """
state = self.history[-1]
return np.sqrt(state.x**2 + state.y**2)
def displacement(self):
""" distance between the starting and ending point """
return np.sqrt((self.history[0].x - self.history[-1].x)**2 +
(self.history[0].y - self.history[-1].y)**2 )
def energy(self, n):
""" return the energy (per unit mass) at time n """
state = self.history[n]
return 0.5 * (state.u**2 + state.v**2) - \
GM / np.sqrt(state.x**2 + state.y**2)
def rhs(self, state):
""" RHS of the equations of motion."""
# current radius
r = np.sqrt(state.x**2 + state.y**2)
# position
xdot = state.u
ydot = state.v
# velocity
udot = -GM * state.x / r**3
vdot = -GM * state.y / r**3
return OrbitState(xdot, ydot, udot, vdot)
def single_step(self, state_old, dt):
""" take a single RK-4 timestep from t to t+dt for the system
ydot = rhs """
# get the RHS at several points
ydot1 = self.rhs(state_old)
state_tmp = state_old + 0.5 * dt * ydot1
ydot2 = self.rhs(state_tmp)
state_tmp = state_old + 0.5 * dt * ydot2
ydot3 = self.rhs(state_tmp)
state_tmp = state_old + dt * ydot3
ydot4 = self.rhs(state_tmp)
# advance
state_new = state_old + (dt / 6.0) * (ydot1 + 2.0 * ydot2 +
2.0 * ydot3 + ydot4)
return state_new
def integrate(self, dt_in, err, tmax):
""" integrate the equations of motion using 4th order R-K method with an
adaptive stepsize, to try to achieve the relative error err. dt
here is the initial timestep
"""
S1 = 0.9
S2 = 4.0
# start with the old timestep
dt_new = dt_in
dt = dt_in
n_reset = 0
t = 0
while t < tmax:
state_old = self.history[-1]
if err > 0.0:
# adaptive stepping iteration loop -- keep trying to take a step
# until we achieve our desired error
rel_error = 1.e10
n_try = 0
while rel_error > err:
dt = dt_new
if t+dt > tmax:
dt = tmax-t
# take 2 half steps
state_tmp = self.single_step(state_old, 0.5*dt)
state_new = self.single_step(state_tmp, 0.5*dt)
# now take just a single step to cover dt
state_single = self.single_step(state_old, dt)
# state_new should be more accurate than state_single since it
# used smaller steps
# estimate the relative error now
rel_error = max(abs((state_new.x - state_single.x) / state_single.x),
abs((state_new.y - state_single.y) / state_single.y),
abs((state_new.u - state_single.u) / state_single.u),
abs((state_new.v - state_single.v) / state_single.v))
# adaptive timestep algorithm for RK4
dt_est = dt * abs(err/rel_error)**0.2
dt_new = min(max(S1*dt_est, dt/S2), S2*dt)
n_try += 1
if n_try > 1:
# n_try = 1 if we took only a single try at the step
n_reset += (n_try-1)
else:
# take just a single step
if t + dt > tmax:
dt = tmax - t
# take just a single step to cover dt
state_new = self.single_step(state_old, dt)
# successful step
t += dt
# store
self.time.append(t)
self.history.append(state_new)
def plot(self, points=False):
"""plot the orbit"""
fig, ax = plt.subplots()
x = [q.x for q in self.history]
y = [q.y for q in self.history]
# draw the Sun
ax.scatter([0], [0], marker=(20,1), color="y", s=250)
if points:
ax.scatter(x, y)
else:
ax.plot(x, y)
ax.set_aspect("equal")
return fig
if __name__ == "__main__":
o = OrbitsRK4(1.0, 0.95)
o.integrate(0.05, 1.e-8, 10.0);
print("number of points = ", o.npts())
# plot the orbit
fig = o.plot()
fig.tight_layout()
fig.savefig("adaptive_rk4_orbit.png", bbox_inches="tight")
E = []
for n in range(o.npts()):
E.append(o.energy(n))
fig, ax = plt.subplots()
ax.plot(o.time, E/E[0])
ax.set_xlabel("time [yr]")
ax.set_ylabel("E/E(t=0)")
fig.savefig("adaptive_rk4_energy.png")
Highly eccentric orbit#
Let’s create an orbit with a tolerance of \(10^{-5}\), and the a very high eccentricity (\(a = 1\), \(e = 0.95\))
o = orbit.OrbitsRK4(1.0, 0.95)
o.integrate(0.05, 1.e-5, o.kepler_period())
How many points did it take?
o.npts()
92
Let’s plot it
fig = o.plot(points=True)
fig.set_size_inches(5, 12)
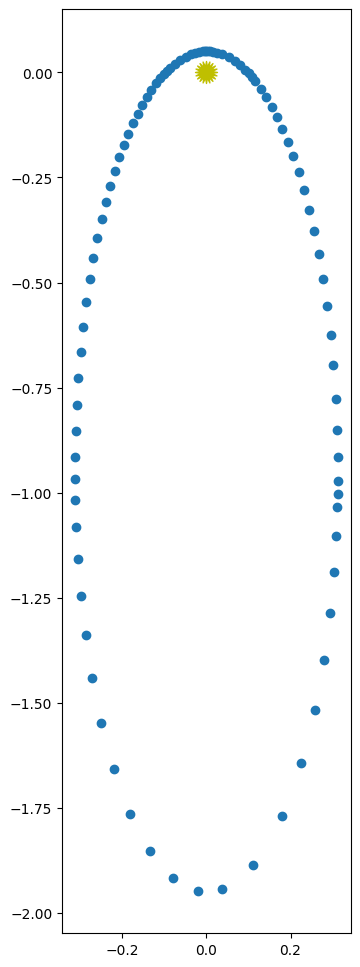
Clearly we see that the size of the timestep is much larger at aphelion as compared to perihelion.
Let’s create a version with a fixed timestep, with a timestep of \(0.001~\mathrm{yr}\). We can do this by passing in a negative error.
o_fixed = orbit.OrbitsRK4(1.0, 0.95)
o_fixed.integrate(0.001, -1, o_fixed.kepler_period())
fig = o_fixed.plot()
fig.set_size_inches(5, 12)
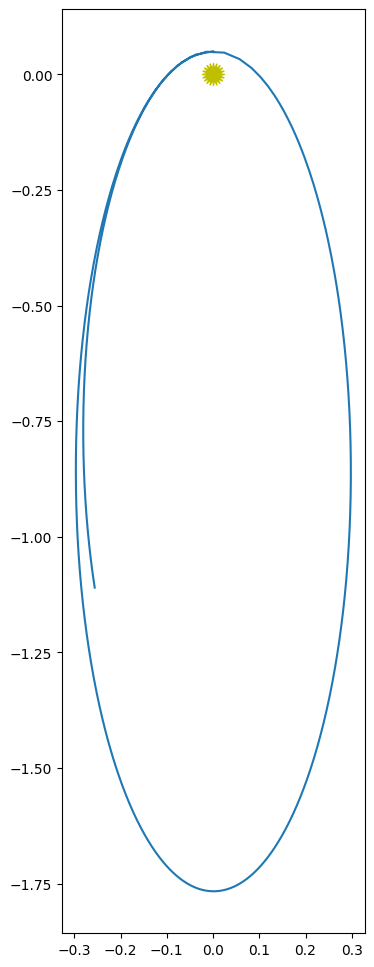
print(o_fixed.npts())
1001
We need a really small fixed step size to integrate this at all reasonably
Let’s look at the adaptive version some more. First, let’s look at the timestep evolution.
ts = np.array(o.time)
dt = ts[1:] - ts[:-1]
tc = 0.5 * (ts[1:] + ts[:-1])
fig, ax = plt.subplots()
ax.plot(tc, dt)
ax.set_xlabel("t [yr]")
ax.set_ylabel("dt [yr]")
ax.set_yscale("log")
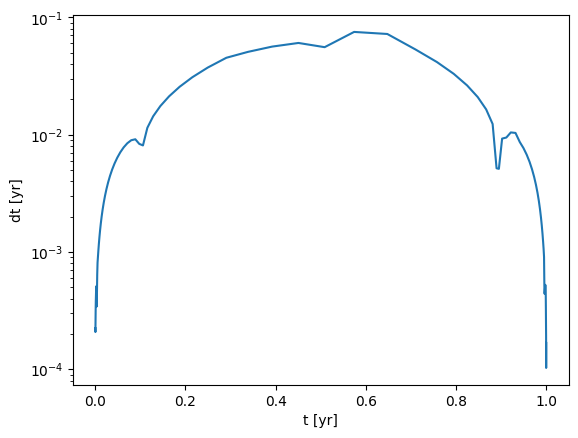
Notice that the timestep changes by ~ 3 orders of magnitude over the evolution.
What about energy conservation?
e = []
ts = o.time
for n in range(o.npts()):
e.append(o.energy(n))
fig, ax = plt.subplots()
ax.plot(ts, e/e[0])
ax.set_xlabel("time [yr]")
ax.set_ylabel("E/E(t=0)")
ax.ticklabel_format(useOffset=False)
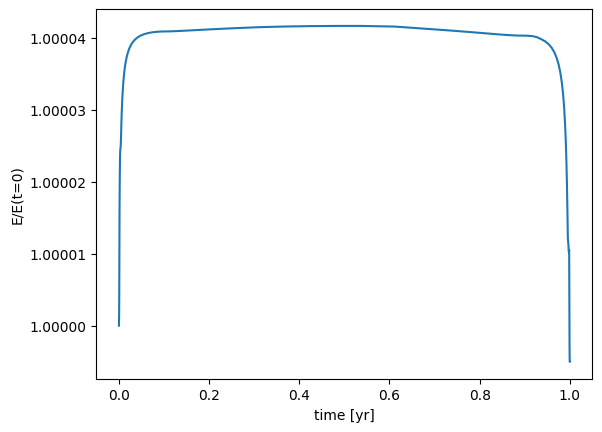
Now let’s do 10 orbits
o = orbit.OrbitsRK4(1.0, 0.95)
o.integrate(0.05, 1.e-5, 20*o.kepler_period())
e = []
ts = o.time
for n in range(o.npts()):
e.append(o.energy(n))
fig, ax = plt.subplots()
ax.plot(ts, e/e[0])
ax.set_xlabel("time [yr]")
ax.set_ylabel("E/E(t=0)")
ax.ticklabel_format(useOffset=False)
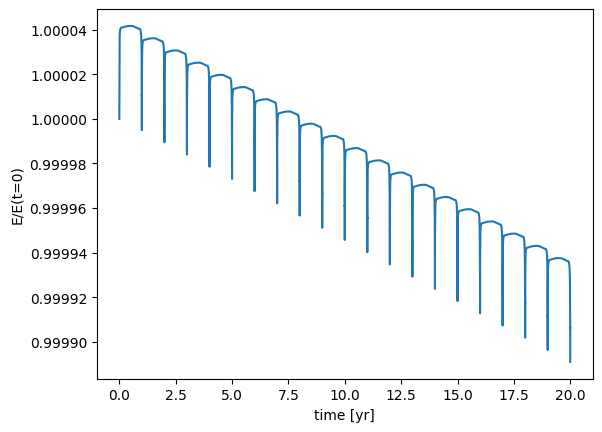
We can see that RK4 does not conserve energy! There is a steady decrease in the total energy over 20 orbits. If we wanted to evolve for millions of years, this would certainly be a problem. Making the tolerance tighter certainly will help, but it will also make things a lot more expensive.
The problem is that 4th order Runge-Kutta does not know anything about energy conservation.